Efficient exploration in crowds by coupling navigation controller and exploration Planner
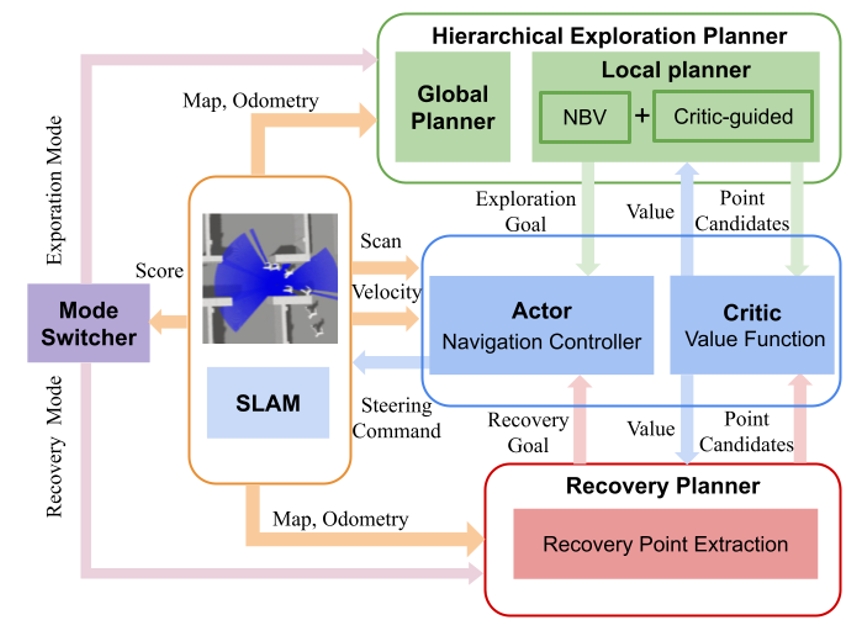
Overview
Autonomous exploration in scenes with moving pedestrians is critical for deploying autonomous mobile robots in populated places such as malls, airports, and museums. The existence of dynamic obstacles poses challenges on achieving an efficient, safe, and robust exploration system: the robot may get stuck in the pedestrians without making progress in scene coverage; it may collide with humans and hurt them; the human-robot collision will fail the exploration process or cause large drift and artifacts in simultaneous localization and mapping (SLAM). In this work, we propose a framework that can solve these challenges by tightly coupling a reinforcement learned navigation controller and a hierarchical exploration planner enhanced with a recovery planner. The navigation controller provides a value function describing the distribution of crowds around the robot, which will be leveraged by exploration planner and recovery planner to minimize the human-robot interruptions. We evaluate the proposed exploration framework against several methods on a set of indoor benchmarks with pedestrians, verifying the advantages of our method in terms of exploration efficiency, navigation safety, and SLAM quality.