Joint prediction of pedestrians and occupancy grid map
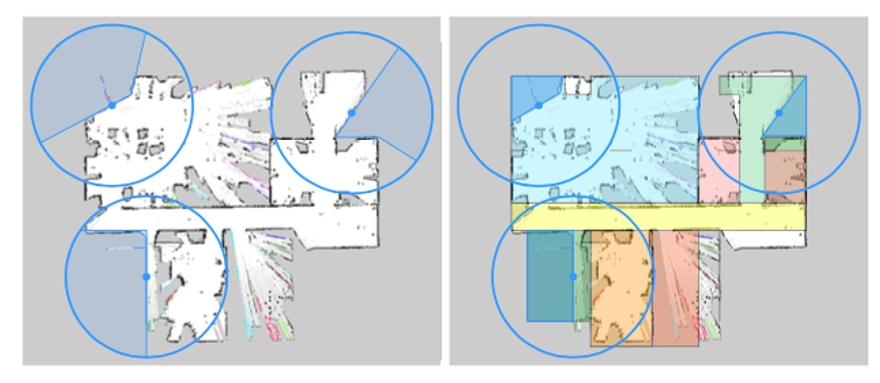
Predictions about maps can aid in the computation of information gain in autonomous exploration tasks, thereby improving exploration efficiency. Various studies have highlighted the favourable influence of precise map prediction on the effectiveness of autonomous exploration tasks.
This project aims to develop a comprehensive framework that combines map prediction and pedestrian tracking, focusing on the interaction between pedestrians and the environment to improve performance in predicting both map and pedestrian behaviours. The joint estimation process follows an iterative approach. The integration of an artificial potential field guides pedestrian motion based on the predicted map, thus improving the accuracy of pedestrian tracking. Conversely, the predicted crowd field, derived from pedestrian tracking and prediction, refines the map prediction process by utilizing image segmentation algorithms.
One key aspect of our approach is the inclusion of a feedback loop in the joint estimation system, allowing for continuous refinement and improvement of both the map prediction and pedestrian tracking components. Through iterative updates and enhancements based on the reciprocal relationship between pedestrians and the environment, our algorithm achieves increased accuracy in predicting various aspects, including the shape and size of the map and the trajectories of pedestrians.
The incorporation of map prediction and pedestrian tracking in our joint estimation framework signifies a substantial breakthrough in autonomous robot exploration. By utilizing the interplay between pedestrians and the environment, our algorithm exhibits favourable outcomes in terms of enhanced prediction accuracy and robustness. This framework has the potential to facilitate more efficient and effective exploration in intricate environments, thus advancing the capabilities of autonomous mobile robots in diverse real-world scenarios.